Research
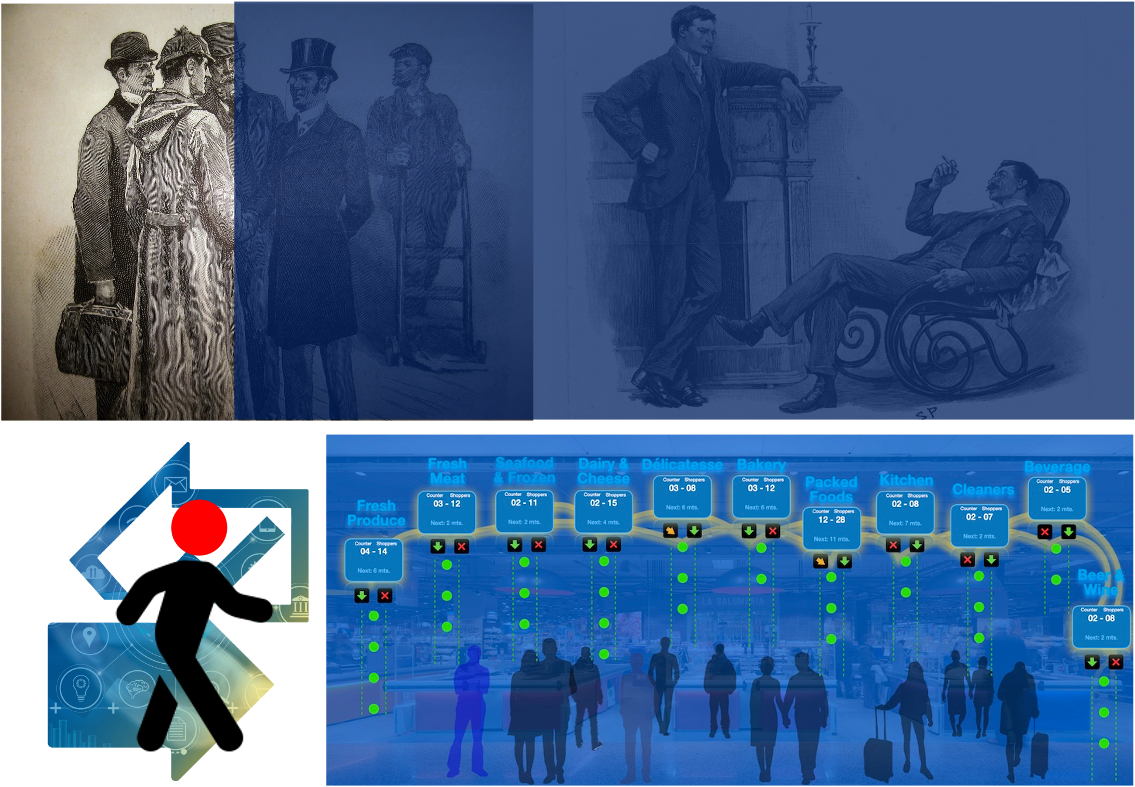
Causal Inferences Project Reward-Driven Insights
Just to set the record, machine learning inference is very different from statistical inference or inference for decision-making in social sciences and business.
In machine learning, inference is the process of running data points into a machine learning model to calculate an output such as a single numerical score. In short, machine learning inference is the ability of a system to make predictions from novel data.
However, statistical inference is about drawing conclusions based on data – historical or new. Statistical inference is all about making projections about what will happen based on statistical analysis of other datasets. In social science we are sometimes in the position of studying descriptive questions (In what places do consumers prefer for Coca-Cola bottles? In what eras has social mobility been higher in the United States than in Europe? In what social settings are different sorts of people more likely to act strategically?). Answering descriptive questions is not easy and involves issues of graph-data measurement, but is uncontroversial from a statistical stand- point. All becomes more difficult when we shift our focus from what to what if and why.
Consider two broad classes of inferential questions:
1. Forward causal inference. What might happen if we do X? What are the effects of smoking on health, the effects of schooling on knowledge, the effect of campaigns on election outcomes, and so forth?
2. Reverse causal inference. What causes Y? Why do more attractive women get rich husbands? Why do many poor people scarce for value-for-money and rich people scarce for time-for-money? Why did the social-security collapse?
Here is an Insight: “Product satisfaction arises less from inherent construction and performance than from consumers’ internalised perceptions of personal utility.”
Between 40 and 50 years ago, Professor Theodore Levitt famously told his Harvard Business School students: “People don’t want quarter-inch drills. They want quarter-inch holes.”
It’s been quoted a million times ever since and enlightened generations of marketing people.
But what if Professor Levitt had chosen to say this: “Product satisfaction arises less from inherent construction and performance than from consumers’ internalised perceptions of personal utility.” (Doesn’t improve with repetition, does it?)
And the difference in value between these two has nothing to do with the intrinsic observation itself. Both assertions contain the same truth. In fact, to be really pedantic, the low-intensity version, when scrutinised carefully, actually turns out to be more accurate and more comprehensive than the Levitt version – because, of course, people don’t want quarter-inch holes any more than they want quarter-inch drills.
There seems to be no universal agreement on what an insight is but a reasonable definition would seem to be something like this:
“A new understanding, probably of human behaviour or attitude, as a result of which action may be taken and an enterprise more efficiently conducted.”
Interestingly, higher order networks have a natural geometric interpretation and therefore constitute a nat- ural way to explore the discrete network geometry of complex networks. Here we investigate the rich interplay between emergent network geometry of higher order networks and their complexity in the framework of a non-equilibrium model
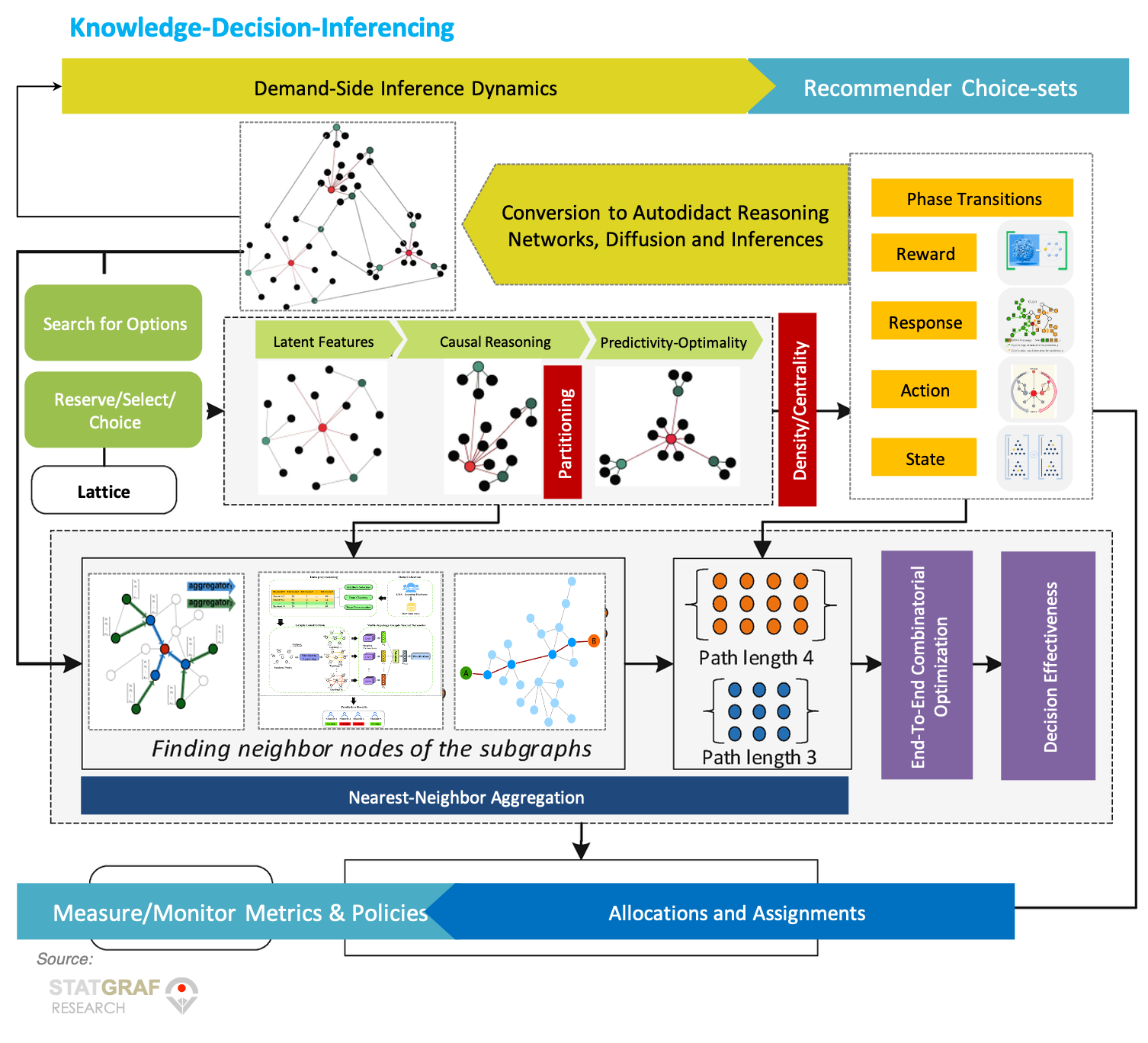
The call for insights is natural. To return to Levitt’s dictum, companies don’t want research; they want enlightenment. Conventional market research, professionally conducted, can paint an invaluable picture of the immediate past; but companies also need help in forging their futures. That’s what lies behind the demand for insights – but not all insights are equal. They come in two very different styles and with very different values.
There are low-potency insights and there are high-potency insights.
In business, we seem to want to follow the linguistic philosophers; to believe that the rigorous researcher or the business professional deals only in matters of fact; always defines terms; and aims for the total elimination of ambiguity.
In fact, of course, if every word employed is underpinned by definition, it follows that every definition employed needs to be underpinned by definition – and so on into what is called infinite regression. In the pursuit of economy and precision, we achieve instead circumlocution, opacity and chaos.

Instead, when searching for high-potency expression of sometimes complex insights, it’s a great deal more fruitful to accept the limitations of language; and to agree with Arthur Koestler when he says that
“Words in themselves are never completely explicit; they are merely stepping stones for thought.” (It’s a wonderful sentence that; not only an important insight, but an elegant example, in itself, of the very truth it contains.)
High-potency insights, because of their immediacy – because they evoke as well as inform – they are infectious; we only have to hear them once to remember them, to apply them, to pass them on to others.
By contrast, the low-potency insight sits there sullenly on its PowerPoint slide, moving absolutely nobody to enlightenment, let alone action.
Every time the consumers buy a brand, select a function or perform an activity, they exercise a choice. These individual choices, in aggregate, flow into demand-density to capacity-diffusion formulations that review volume flow and speed or velocity of functional metrics around such choices and, thereby, option-linked recommendations are directed for single and multiple decisions in a dynamic environment. These also review the use of multi-disciplinary-multi-stakeholder perspectives to understand the interlinks between high-frequency throughput and ultra-low latency for impact on consumers’ experience.
Salience (also called saliency) is that property by which something stands out. Salient events are an attention mechanism by which organisms learn and survive; those organisms focus their limited perceptual and cognitive resources on the pertinent (that is, salient) subset of the sensory data available to them. Saliency typically arises from contrasts between items and their neighborhood. They might be represented, for example, by a red dot surrounded by white dots, or by a flickering message indicator of an answering machine, or a loud noise in an otherwise quiet environment.
A saliency mapping is a collection of cues in a context that highlights the region on which people focus and make their choices. The goal of a saliency map is to reflect the degree of importance of underlying dimensions to the human cognitive system.