Research
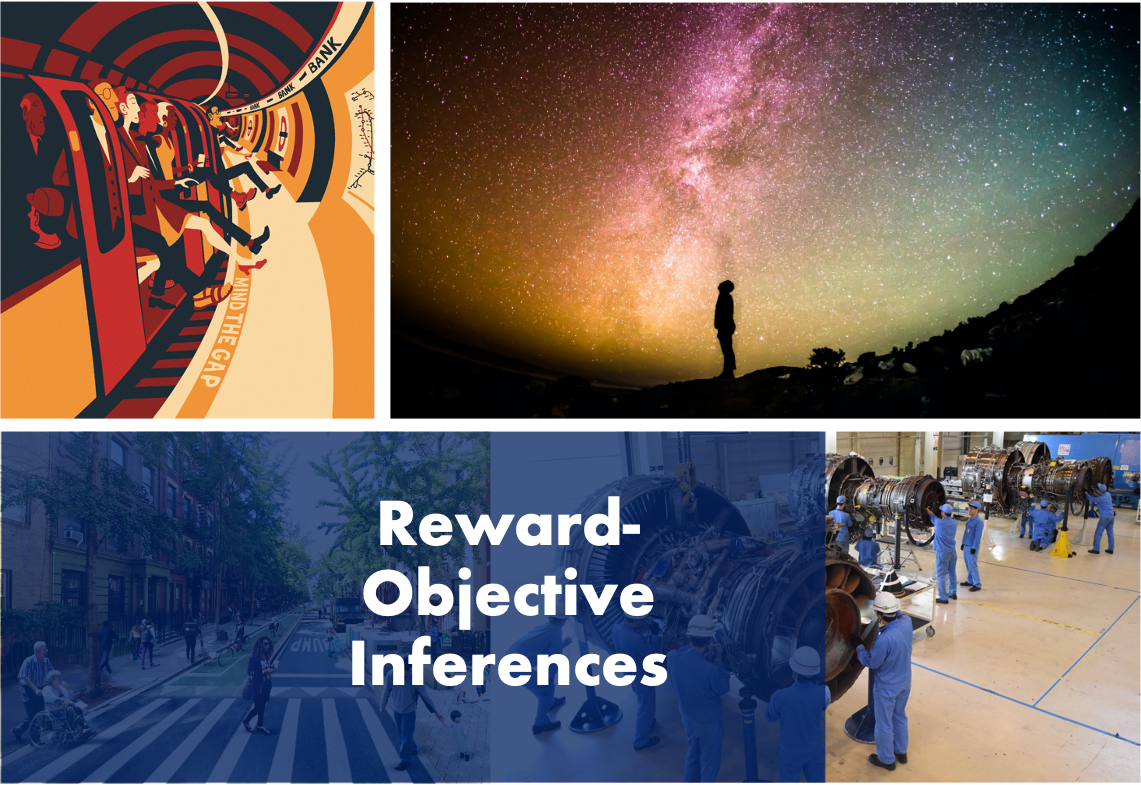
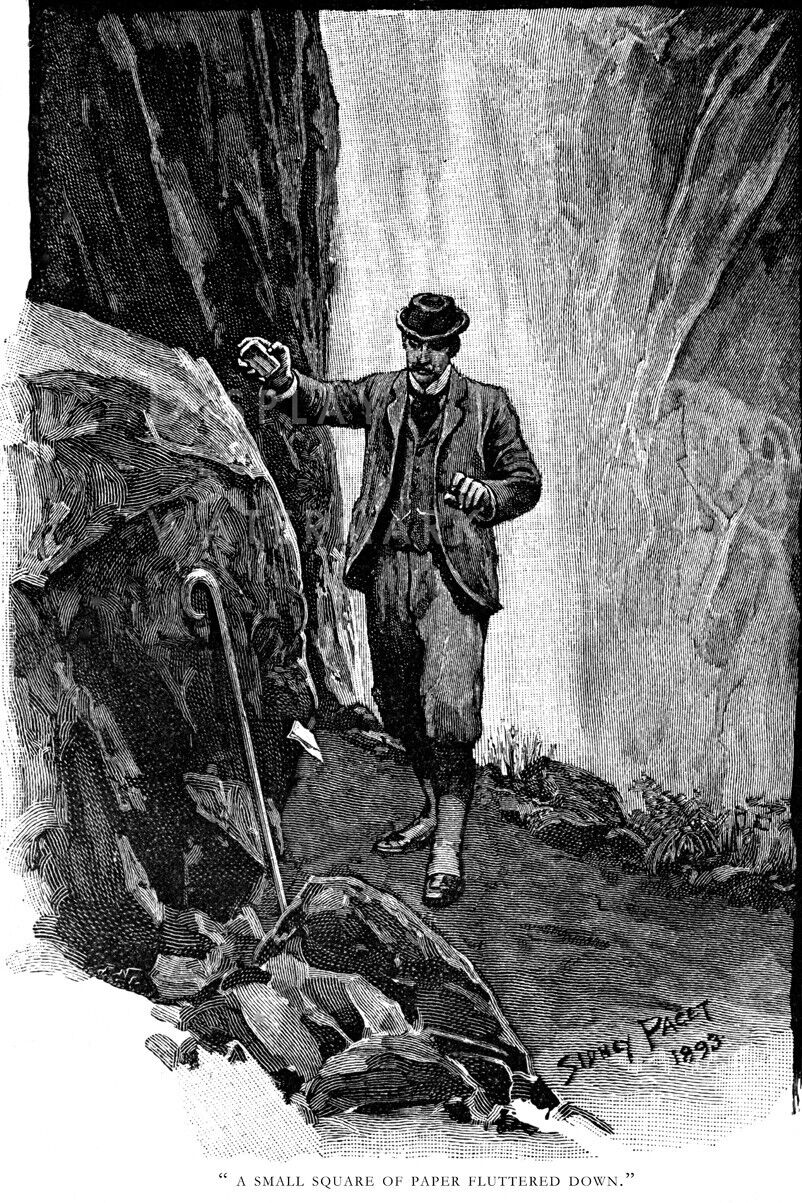
Holmes is the cold, rational problem-solver who thinks laterally and remembers only what is useful to him, Watson the warm, intelligent everyman who thinks linearly and unimaginatively. We are astonished by Holmes’s powers and infuriated by his arrogance but glad that he is around to put the world to rights … He brings order to muddle.
“It was most suggestive,” said Holmes. “It has long been an axiom of mine that the little things are infinitely the most important.” One of the key factors that set Holmes apart was his incredible attention to detail. He had a remarkable ability to observe even the most minute aspects of a crime scene, often noticing things that others would overlook. Whether it was a misplaced hair or a smudge on a windowpane, Holmes understood that every clue, no matter how seemingly insignificant, could hold the key to solving the mystery.
Another thing we can learn from Holmes is the importance of continuous self-education. When Watson asks why he persists in pursuing a case that seems solved, Holmes replies: “It is art for art’s sake. I suppose when you doctored you found yourself studying cases without a thought of a fee?” Watson answers, “For my education, Holmes.” Just so, Holmes replies. “Education never ends.”
The reasoning and inferencing model ensembles (RIME) has used thousands of audio, video, cognitive and context data, public and/or proprietary, to train a set of models to forecast actions; optimize reward function; derive salient choice-sets, minimize loss functions, etc. on the following objectives:
Recognition
Experiential
Decomposability
Optimization
Networks
Diffusion
In the context where dimensionality is an issue, we have experimented that macroscopic behaviors are typically well described by the theoretical limit of infinitely large systems. Under this limit, the statistical physics of disordered systems offers powerful frameworks of approximation called mean-field theories. Interactions between physics, operational research and neural network already have a long history. Yet, interconnections have been re-heightened by the recent progress in learning technologies, which also brought new challenges. The goal of SCANN learning is to directly extract structure from data.
The training dataset were real-world data-sets and not synthetic data. So, they are made of a set of example inputs D = {x }P without corresponding outputs. The SCANN learning algorithms either implicitly or explicitly adopt a probabilistic viewpoint and implement density estimation. The idea is to approximate the true density from which the training data was sampled by the closest element among a family of parametrized distributions of the input space.
Here, we wish to provide a concise methodological review of fundamental mean-field inference methods with their application to neural networks for decision-making in mind. Our aim is also to provide a unified presentation of the different approximations allowing to understand how they relate and differ. Methods presented in the reference have a significant overlap with what will be covered in terms of application as a product, service and platform. The approximations and algorithms that discussed here are also largely peer-reviewed. This includes more details on spin glass theory, which originally motivated the development of the classical mean-field methods, and particularly focuses on community detection and linear estimation. Despite the significant overlap and beyond their differing motivational applications, the two previous references are also anterior to some recent exciting developments in mean-field inference covered in the present review, in particular extensions toward multi-layer networks. An older, yet very interesting, reference is the client-engagement proceedings, which collected both insightful introductory papers and research developments for the applications of mean-field methods in machine learning. Finally, the recent covers more generally the connections between physical sciences, operations research and machine learning yet without detailing the methodologies. This review provides a very good list of references where statistical physics methods were used for learning theory, but also where machine learning helped in turn physics research.
Observing Sherlock Holmes in his adventures no doubt facilitates the understanding of the phenomenon of case-based reasoning, where one refers to a previous situation in order to adapt it to a new situation. Sherlock Holmes takes the standpoint that ‘there is a strong family resemblance about misdeeds, and if you have all the detail of a thousand at your finger ends, it is odd if you can’t unravel the thousand and first,’ and thus often tackles cases by remembering and adapting past inquiries: ‘It reminds me of the circumstances attendant on the death of Van Jansen, in Utrecht, in the year ‘34.’
[A Study in Scarlet (1887)]