Research
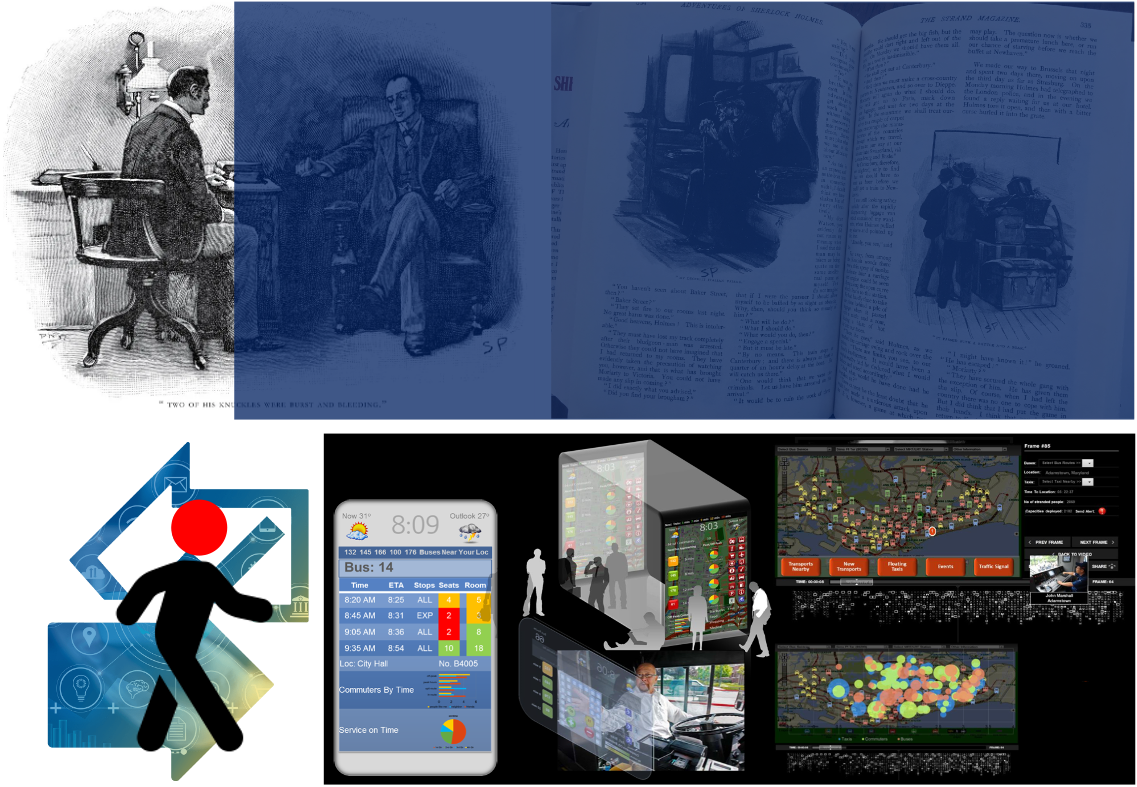
Decomposability
Decomposability or near-decomposability is organized, in setting of Fosbury-Flop into layers of work and effort or parts, parts of parts, parts of parts of parts and so on, in such a way that learning model is set for interactions among elements belonging to the same parts are much more than interactions among elements belonging to different parts.
If we go back to the late 1960’s when Dick Fosbury discovered the Fosbury Flop high jump technique, we find the reason for his unique jumping style.
From a physics standpoint, To do this the athlete begins an approach a certain distance from the high jump bar, usually between 40 and 60 feet in front, and 10-14 feet to the side of the bar. The athlete first runs straight forward, building horizontal speed, then follows a curve towards the bar. The curve helps the athlete reach the proper take-off position, in which the person is leaning slightly away from the bar facing the back-left corner of the landing area (if they are approaching from the same side). Once the athlete reaches the take-off point, he or she plants their foot and uses their slight lean away from the bar to convert their horizontal velocity into both vertical velocity and angular momentum. The vertical velocity helps raise the center of mass of the athlete, while the angular momentum helps rotate the athlete’s body into the position with accurate timing – by arching his back and curling his legs around the bar, his center of mass could pass under the bar, while his body passed over it. Thus, he didn’t need to jump as high to clear the bar, which is much more efficient.
This was a genuine revolution in high jump technique as well as track and field.
Everything that happens in the approach is setting-up the partition functions in learning to be in the correct position at each part before takeoff. It’s the main factor that determines how successful bar clearance. Once one gets the parts and parts of parts right, the probability of making higher heights skyrockets!
Reward-Objective Inference
We compiled thousands of audio-video data on high-jump – from practice session to local events to Olympics – in hundreds of different partition sequences to train machine learn partitioning and work-breakdown into smaller decisions. It became clear that the bar clearance was not the only difference between the “standard” dive straddle and the Fosbury-flop: Fosbury’s run-up was curved, and his arm and lead leg actions during the takeoff phase were weaker than in the straddle. The day after the Mexico high jump final, practically every high jumper in the world tried the new technique. They readily adopted Fosbury’s curved run-up (even though they did not know why-or if- the curve was needed), but most of them added a double-arm action and a straight lead leg, since these were regarded as basic elements for any high jumping technique. However, this did not work: They found it impossible to attain at the peak of the jump the desired face-up position, perpendicular to the bar.
There were several patterns about the curved run-up. The curve allowed the athlete to use force to generate more lift. The curve also allowed the athlete to start during the run-up the rotation needed for the bar clearance that allows the athlete to concentrate exclusively on the generation of lift during the takeoff. The learning model, according to previous research, was set to learn all the angular momentum in a Fosbury-flop that is generated during the takeoff phase and technique in physics looking at the point-particle system, which allows machine to see the underlying motion of a system, and not during the run-up. The curve was useful in two ways: (a) It allowed the athlete to be in a low position at the end of the run-up without having to run with very bent knees; (b) the curve made the athlete lean away from the bar at the time that the takeoff foot was planted, and this permitted the generation of angular momentum during the takeoff without having to lean into the bar by the end of the takeoff.

The goal is to understand how machines partition a task – based on combination of different attributes and features and estimate the right utility – to achieve desired results at each partition.
The goal is to understand in what conditions machine may fail because the state features were insufficient to make accurate predictions, different task objectives defining the reward function may be imbalanced, the agent may fail to sufficiently explore the state-action space, values may not accurately propagate to more distant states, the neural network may not have sufficient capacity to approximate the policy or value function(s), or, there may be subtle differences between training and evaluation environments. Without a way to diagnose the causes of poor performance or to recognize when a problem has been remedied, practitioners typically engage in a long trial-and-error design process until an agent reaches a desired level of performance.

Multi-modal knowledge representation learning takes a high-dimensional feature vector as input and produces a prediction or classification score as output. Functional decomposition is an interpretation technique that deconstructs the high-dimensional function and expresses it as a sum of individual feature effects and interaction effects that can be visualized. Our SCANN architecture in cognitive framework – based on partition functions, states-action-response-reward functions of the target and contexts was configured to predictive control process so that each partition functional decomposition is a fundamental principle underlying many interpretation techniques – it helps understand other interpretation methods. This separates a reward function into distinct components and learns value estimates for each. These value estimates provide insight into an agent’s learning and decision-making process and enable new training methods to mitigate common problems.
Frequency of Interactions: determines the decomposability within a subunit have closer, more widespread, more intense and more frequent interactions than individuals belonging to different subunits
Near-Independence: determines subunits with kinds of interdependencies in its activities will benefit from less-frequent interaction